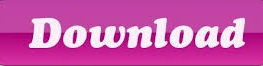
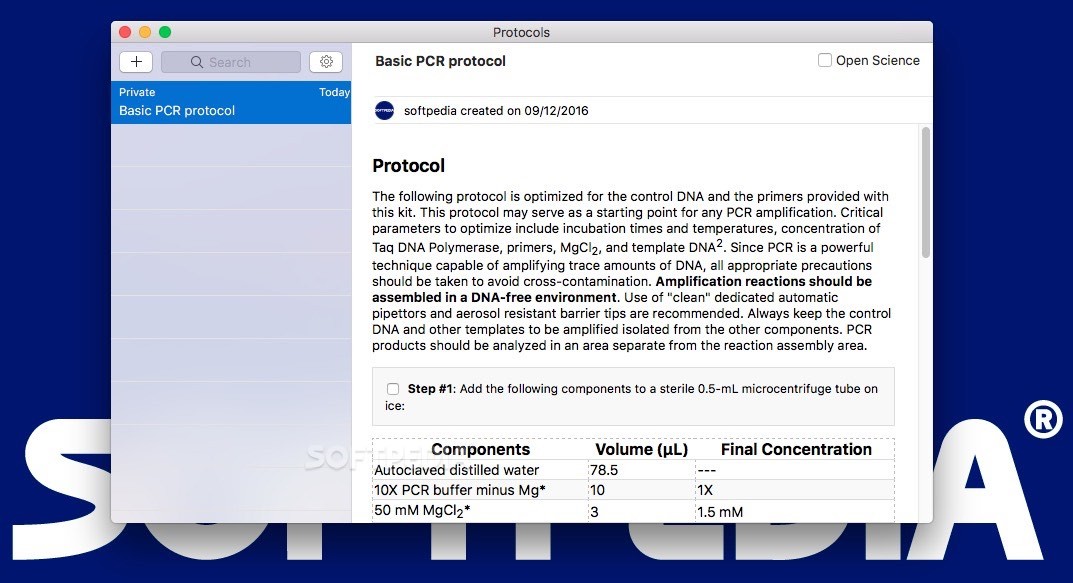
We understand how important it is for institutions to protect their data, and as such, private data needs to stay private with users and institutions controlling who gets to access data and when. Researchers should be able to take ownership of their data. Any lab notebook tool (ELN tool) will help to annotate the data it is important that these tools do not stand by themselves but are integrated into the broader data ecosystem. One of the reasons we saw the Mendeley Data notebook tool Hivebench as an essential component in our research data management platform was that it helps researchers keep very structure datasets, which they can then be shared in a way that’s standardized and comprehensible. Data provenance is crucial for comprehension. Data needs to be comprehensible.įor data to be reused, it needs to be clear which units of measurements were used, how the data was collected, and which abbreviations and parameters are used. Related to that is the idea of comprehensibility. However, this process has a limit: we believe data platforms should automatically and dynamically enrich the metadata using AI techniques to improve discoverability over a longer period of time. The starting point here is that we expect that researchers and the data community have good intentions to add high-quality metadata to the research data. That can mean the data is easily found in search results or when stored in institutional repositories or publisher platforms, or that it’s presented by recommendation engines according to parameters set by researchers. To truly unlock the potential of research data, it needs to be discoverable.
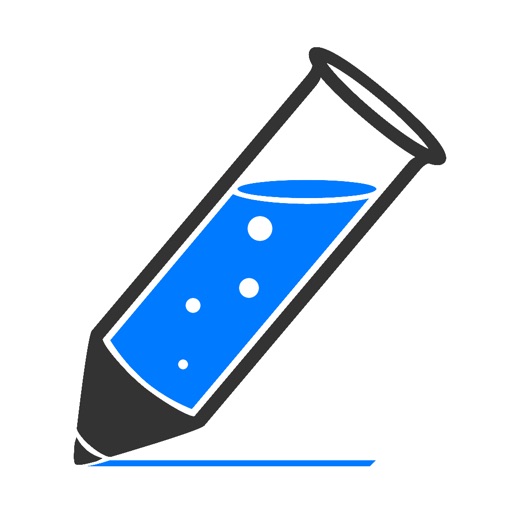
Data needs to be discoverable.Īs with all research outputs, data that’s accessible is limited in value.
PUT DOCUMENTS IN HIVEBENCH FULL
4 principles for unlocking the full potential of research data (Mendeley Data) 1.
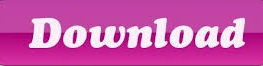